MBTP Symposium Speakers present on Biotechnology and Machine Learning
The annual Molecular Biology Training Program (MBTP) symposium is a place for professors, graduate students, and undergraduates to network and talk about research. Due to the profoundly saddening events within NC State’s community over the last weeks, Chancellor Randy Woodson called for a wellness day on the same day the in-person student symposium was planned. University wellness days call for no classes and are implemented as an opportunity for students to take a break and prioritize their mental health. In the spirit of this, the student portion of the MBTP symposium was postponed. The MBTP program is planning for the in-person poster session to be held during the upcoming spring semester.
The external guest speakers and panel discussion were held on Wednesday, November 9th on Zoom, as originally planned. Dr. Albert Keung, head of the Keung lab, hosted the online event whose theme was “Biotechnology and Machine Learning.” This is a field that I, a somewhat technologically-challenged undergraduate student, am very unfamiliar with. Despite this, I joined the Zoom meeting with a glimpse of hope that I would be able to follow along with the speakers.
Dr. Hal Alper, Kenneth A. Kobe Professor of Chemical Engineering at the University of Texas at Austin and Executive Director for the Center for Biomedical Research Support, was the first speaker to present. Dr. Keung started with a brief introduction, mentioning how well-rounded a scientist Dr. Alper is. “One of the things that is really impressive about Dr. Alper is his strong versatility across many different areas [of science and engineering]” he said. Right off the bat, I noticed that not only is Dr. Alper a great scientist, but also a great presenter. He began his presentation by saying, “I wanted to tell a little story on how we were able to combine the areas of protein engineering, synthetic biology, and machine learning with our overall desire to generate sustainable types of solutions for the environment.”
Titled “Machine learning-aided engineering of PET degrading enzymes,” Dr. Alper’s presentation illustrated how his lab took the PETase enzyme naturally found in Ideonella sakaiensis and engineered it to create FAST-PETase. Their enzyme was created to increase stability while still maintaining efficient degradation of PET, or polyethylene terephthalate. PET is a highly used and recognizable plastic; it’s clear, sturdy, and recyclable. You probably have something made of this material in your sight as you’re reading this. However, not all PET gets recycled, and it’s not biodegradable otherwise. The Alper Lab’s engineered FAST-PETase, which is used by microbes to degrade PET plastics, is highly desired with today’s plastic consumption levels.
The second speaker was Dr. Beth Cimini, head of the Cimini Lab and Senior Group Leader of the Imaging Platform at the Broad Institute of MIT and Harvard. Dr. Keung said, “In a very short amount of time she’s established and driven through multiple collaborative projects across diverse experimental systems,” while introducing her and her presentation, which was titled “Making the most of your high content imaging using machine learning.” Dr. Cimini immediately grabbed my attention by commenting on how cell images are not only pretty but also super informative when paired with the right software, elaborating on how “images are really a quantitative data source.”
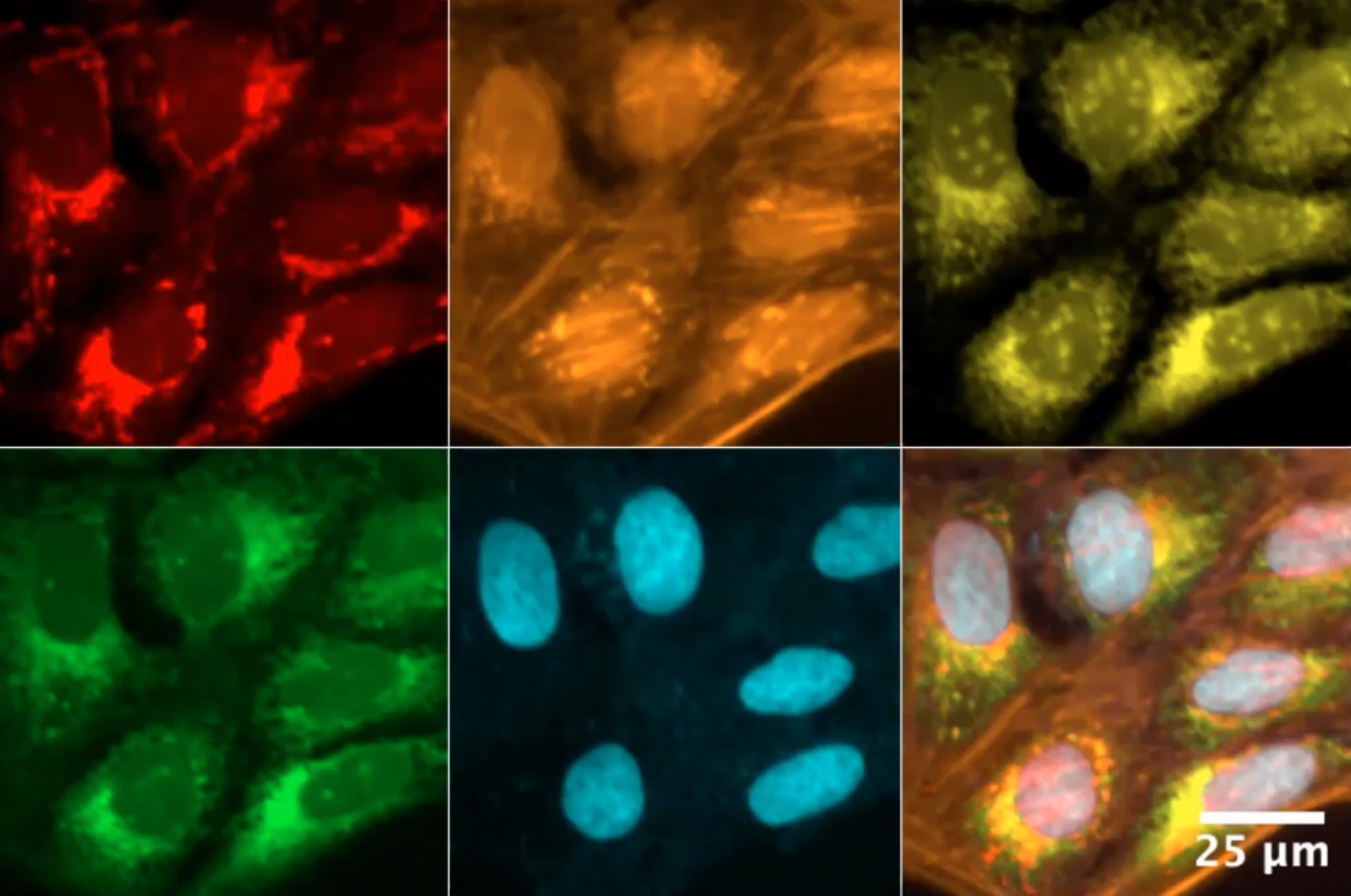
Dr. Cimini highlighted three main ways to use machine learning in conjunction with images. The first method is through segmentation, which is where you want the software to be able to recognize exactly what it’s looking at. The second method is called “feature extraction,” where the software can use deep learning to produce measurements from images. Deep learning, a concept I was unfamiliar with, is a really important aspect of machine learning; it’s the ability of artificial intelligence (AI) to learn similarly to how a human would. Feature extraction is the third method of using machine learning with images and is also referred to as classification, which is exactly as it sounds. Dr. Cimini emphasized how biologists love to classify and organize what they find, and machine learning has the capability of making that so much easier.
Dr. Harmen Bussemaker, the third and final presenter, is a professor of Biological Sciences and Systems Biology at Columbia University. His research group focuses on developing new tools and using deep sequencing techniques to understand human aging and longevity. His presentation, “Probing the DNA binding specificity of transcription factors with deep sequencing and biophysically interpretable machine learning” is quite a mouthful but is easier to digest when broken up and recognizing Dr. Bussemakers background in physics and biochemistry. Formerly trained as a theoretical physicist, Dr. Bussemaker has a lot to bring to the molecular biology field both in and out of machine learning.
DNA binding sites are locations within the DNA that transcription factors and other DNA binding proteins can recognize and then bind to. However, some are stronger than others, and for a long time, scientists have written off the weaker binding sites as unimportant. Dr. Bussemaker has discovered how to use deep learning to identify these low-affinity binding sites and show that they are still functional. He and his team developed SELEX-seq, a machine learning tool used to determine the affinity of any protein complex to any DNA binding site.
“Research is rarely linear, and rarely is it fraught with success”
-Dr. Hal Alper
The last 30 minutes of the symposium functioned as a career panel featuring the presenters. This was a refreshing change of pace where undergraduates and graduate students alike were able to ask questions not only about science but also about the applications within machine learning. One of the questions touched on the holistic approach to careers in this field, to which Dr. Alper said, “Research is rarely linear, and rarely is it fraught with success.” No two person’s paths look the same, but by trying more disciplines and narrowing interests, success becomes a lot more attainable. Dr. Bussemaker also chimed in saying, “If you go deeply in one particular path, as you get into the problem, it’ll give you an experience that you can generalize.” This is a really important point to keep in mind for biotechnology. There’s no way you can gain experience in everything the field has to offer before getting a job, but once you’ve learned standard operating procedures in one lab, chances are you will be able to catch on quickly and have applicable skills you can carry with you to other labs.
Another question asked how students should apply their knowledge in the fields of machine learning and AI. Biology is often accompanied by wet lab skills and less with computational skills, so it seems like it would be a hard transition to make. Dr. Cimini, whose lab takes in students from both backgrounds and works with them in a completely dry-lab environment, said, “Connecting [the model] back to the biological question is crucial.” Dr. Alper and Dr. Bussemaker both agreed with this, adding how collaboration is key and having people who understand both wet and dry labs, in addition to old and new techniques/technologies, is really useful for identifying the best way to solve a biological problem.
Although it meant having to shift the student presentations to next semester, honoring the university wellness day was a necessary change the MBTP symposium made in order to prioritize student mental health. The distinguished speakers did an amazing job presenting machine learning’s applications in biology and talking students through the ever-growing field. The graduate student poster session will be held in the Spring of 2023. Keep an eye on this page for when the date is released if you want to learn more about student work!
- Categories: